Reference evapotranspiration prediction using Artificial Neural Networks
DOI:
https://doi.org/10.29059/cienciauat.v17i2.1708Keywords:
neural networks, evapotranspiration, FFNN, ERNN, NARXAbstract
Reference evapotranspiration (ETo) is a hydrological variable of great importance in irrigation management. Its estimation is carried out with the Penman-Montieth (PM) equation that requires many meteorological variables and that are sometimes not available. Since ETo is a nonlinear and complex variable, in recent years alternative methods have emerged for its estimation, such as artificial neural networks (ANN). The objective of this work was to estimate the reference evapotranspiration (ETo) using the Penman-Montieth equation, in order to develop artificial neural network (ANN) models that allow ETo to be predicted in regions with limited climatological information, and in turn to compare the performance of three RNA models: FFNN, ERNN and NARX. Daily informtion was used during the January 1, 2007 to December 31, 2018 period, for the ENP8 and ENP4 meteorological stations in Mexico city. Based on the correlation analysis and the Garson sensitivity analysis, 2 cases were studied for the 3 ANN models: 1) ANN with 6 inputs: solar radiation (Rad), maximum and minimum temperature (Tmax, Tmin), maximum and minimum relative humidity (RHmax, RHmin), and wind speed (u), and 2) RNA with 2 inputs (Rad and Tmax). The output variable was the ETo, calculated with the PM equation. In all cases, the performance of the 3 ANNs was very similar. The most notable difference is that the dynamic networks (ERNN and NARX) require fewer iterations to achieve the optimum performance. ANNs trained only with radiation and maximum temperature as inputs were able to predict a long-term ETo for 440 at another nearby meteorological station (ENP4), with efficiencies greater than 90 %.
References
Abrishami, N., Sepaskhah, A. R., and Shahrokhnia, M. H. (2018). Estimating wheat and maize daily evapotranspiration using artificial neural network. Theoretical and Applied Climatology. 135(3): 945-958. DOI: https://doi.org/10.1007/s00704-018-2418-4
Allen, G. R., Pereira, L. S., Raes, D., and Smith, M. (1998). Crop evapotranspiration - Guidelines for computing crop water requirements - FAO Irrigation and drainage paper 56. Fao. Rome. 300(9): D05109.
Antonio, F. H., Salazar, M. R., Fitz, R. E., López, C. I., Schmidt, U. y Dannelh, D. (2023). Rendimientos y eficiencia en el uso del agua de lechuga y tomate cherry en jardines urbanos. Revista Tecnología y Ciencias del Agua. 14(5): in press. DOI: https://doi.org/10.24850/j-tyca-14-05-05
Bianchi, F. M., Maiorino, E., Kampffmeyer, M. C., Rizzi, A., and Jenssen, R. (2017). Recurrent neural networks for short-term load forecasting: an overview and comparative analysis. Editorial Springer. 74 Pp. DOI: https://doi.org/10.1007/978-3-319-70338-1
Boussaada, Z., Curea, O., Remaci, A., Camblong, H., and Mrabet-Bellaaj, N. (2018). A Nonlinear Autoregressive Exogenous (NARX) Neural Network Model for the Prediction of the Daily Direct Solar Radiation. Energies. 11(3): 620. DOI: https://doi.org/10.3390/en11030620
Diaconescu, E. (2008). The use of NARX neural networks to predict chaotic time series. Wseas Transactions on Computer Research. 3(3): 182-191.
Elman, J. L. (1990). Finding structure in time. Cognitive Science. 14(2): 179-211. DOI: https://doi.org/10.1016/0364-0213(90)90002-E
Gao, S., Zhang, Y., Zhang, Y., and Zhang, G. (2021). Elman neural network soft-sensor model of pvc polymerization process optimized by chaos beetle antennae search algorithm. IEEE Sensors Journal. 21(3): 3544-3551.
García, M. H., Flores, M. H., Ascencio, H. R., Khalil, G. A., Tijerina, C. L., Mancilla, V. O. R., and Vázquez, P. M. A. (2020). Corn grain yield estimation from vegetation indices, canopy cover, plant density, and a neural network using multispectral and RGB images acquired with unmanned aerial vehicles. Agriculture. 10(7): 277. DOI: https://doi.org/10.3390/agriculture10070277
Ghose, D., Das, U., and Roy, P. (2018). Modeling response of runoff and evapotranspiration for predicting water table depth in arid region using dynamic recurrent neural network. Groundwater for Sustainable Development. 6: 263–269. DOI: https://doi.org/10.1016/j.gsd.2018.01.007
González, C. J. M., Cervantes, O. R., Ojeda, B. W. y López, C. I. (2008). Predicción de la evapotranspiración de referencia mediante redes neuronales artificiales. Ingeniería Hidráulica en México. 18(1): 127-138.
Gordillo, S. V. M., Flores, M. H., Tijerina, C. L. y Arteaga R. R. (2014). Estimación de la evapotranspiración utilizando un balance de energía e imágenes satelitales. Revista Mexicana de Ciencias Agrícolas. 5(1): 143-155. DOI: https://doi.org/10.29312/remexca.v5i1.1017
Heng, L. K., Hsiao, T., Evett, S., Howell, T., and Steduto, P. (2009). Validating the FAO AquaCrop model for irrigated and water deficient field maize. Agronomy Journal. 101(3): 488-498. DOI: https://doi.org/10.2134/agronj2008.0029xs
Huo, Z., Feng, S., Kang, S., and Dai, X. (2012). Artificial neural network models for reference evapotranspiration in an arid area of northwest China. Journal of Arid Environments. (82): 81-90. DOI: https://doi.org/10.1016/j.jaridenv.2012.01.016
Instituto de Ciencias de la Atmósfera y Cambio Climático (2018). Programa de Estaciones Meteorológicas del Bachillerato Universitario (PEMBU). [En línea]. Disponible en: https://www.ruoa.unam.mx/pembu/. Fecha de consulta: 13 de septiembre de 2022.
Jahani, B. and Mohammadi, B. (2019). A comparison between the application of empirical and ANN methods for estimation of daily global solar radiation in Iran. Theoretical and Applied Climatology. 137(1-2): 1257-1269. DOI: https://doi.org/10.1007/s00704-018-2666-3
Kisi, Ö. (2006). Generalized regression neural networks for evapotranspiration modelling. Hydrological Sciences Journal. 51(6): 1092–1105. DOI: https://doi.org/10.1623/hysj.51.6.1092
Kumar, M., Raghuwanshi, N. S., Singh, R., Wallender, W. W., and Pruitt, W. O. (2002). Estimating evapotranspiration using artificial neural network. Journal of Irrigation and Drainage Engineering. 128(4): 224-233. DOI: https://doi.org/10.1061/(ASCE)0733-9437(2002)128:4(224)
Kůrková, V. (1992). Kolmogorov’s theorem and multilayer neural networks. Neural Networks. 5(3): 501-506. DOI: https://doi.org/10.1016/0893-6080(92)90012-8
Masters, T. (1993). Practical neural network recipes in C. USA. Academic Press. 493 Pp. DOI: https://doi.org/10.1016/B978-0-08-051433-8.50017-3
Meneses, K. C. D., Aparecido, L. E. D. O., Meneses, K. C. D., and Farias, M. F. D. (2020). Estimating potential evapotranspiration in maranhão state using artificial neural networks. Revista Brasileira de Meteorologia. 35(4): 675-682. DOI: https://doi.org/10.1590/0102-77863540072
Moghaddamnia, A., Ghafari-Gousheh, M., Piri, J., Amin, S., and Han, D. (2009). Evaporation estimation using artificial neural networks and adaptive neuro-fuzzy inference system techniques. Advan Water Resou (32): 88–97. DOI: https://doi.org/10.1016/j.advwatres.2008.10.005
Proias, G., Gravalos I., Papageorgiou, E., Poczęta, K., and Sakellariou-Makrantonaki, M. (2022). Forecasting reference evapotranspiration using time lagged recurrent neural network. WSEAS Transactions on Environment and Development. 16: 699-707. DOI: https://doi.org/10.37394/232015.2020.16.72
Punto Periferia (2021). Ciudad de México: la segunda ciudad con más huertos urbanos en Latinoamérica, en ArchDaily México. [En línea]. Disponible en: https://www.archdaily.mx/mx/963873/ciudad-de-mexico-la-segunda-ciudad-con-mas-huertos-urbanos-en-latinoamerica. Fecha de consulta: 8 de diciembre de 2022.
Rahimi-Khoob, A. (2008). Artificial neural network estimation of reference evapotranspiration from pan evaporation in a semi-arid environment. Irrigation Science. 27(1): 35-39. DOI: https://doi.org/10.1007/s00271-008-0119-y
Ruiz, A. O., Martínez, D. G., Corrales, S. A., González, J. L. A. y Galindo, R. M. A. (2015). La evapotranspiración de referencia en la república mexicana: tres años de 440 evaluación. Reporte No. 64.
Sudheer, K. P., Gosain, A. K., and Ramasastri, K. S. (2003). Estimating actual evapotranspiration from limited climatic data using neural computing technique. Journal of Irrigation & Drainage Engineering. 129(3): 214-218. DOI: https://doi.org/10.1061/(ASCE)0733-9437(2003)129:3(214)
Walls, S., Binns, A. D., Levison, J., and MacRitchie, S. (2020). Prediction of actual evapotranspiration by artificial neural network models using data from a bowen ratio energy balance station. Neural Computing and Applications. 32(17): 14001-14018. DOI: https://doi.org/10.1007/s00521-020-04800-2
Yohanani, E., Frisch, A., Lukyanov, V., Cohen, S., Teitel, M., and Tanny, J. (2022). Estimating evapotranspiration of screenhouse banana plantations using artificial neural network and multiple linear regression models. Water. 14(7): 1130-1130. DOI: https://doi.org/10.3390/w14071130
Yun, K., Qiang, H., and Ma, Y. (2021). Construction of Network Security Perception System Using Elman Neural Network. In 2021 2nd International Conference on Computer Communication and Network Security (CCNS) (pp. 187-190). IEEE. DOI: https://doi.org/10.1109/CCNS53852.2021.00042
Zanetti, S. S., Sousa, E. F., Oliveira, V. P., Almeida, F. T., and Bernardo, S. (2007). Estimating evapotranspiration using artificial neural network and minimum climatological data. Revista de Ingeniería de Riego y Drenaje. 133(2): 83-89. DOI: https://doi.org/10.1061/(ASCE)0733-9437(2007)133:2(83)
Zhao, L., Zhao, X., Pan, X., Shi, Y., Qiu, Z., Li, X., ..., and Bai, J. (2022). Prediction of daily reference crop evapotranspiration in different Chines eclimate zones: combined application of key meteorological factors and elman algorithm. Journal of Hydrology. 610: 1-18. DOI: https://doi.org/10.1016/j.jhydrol.2022.127822
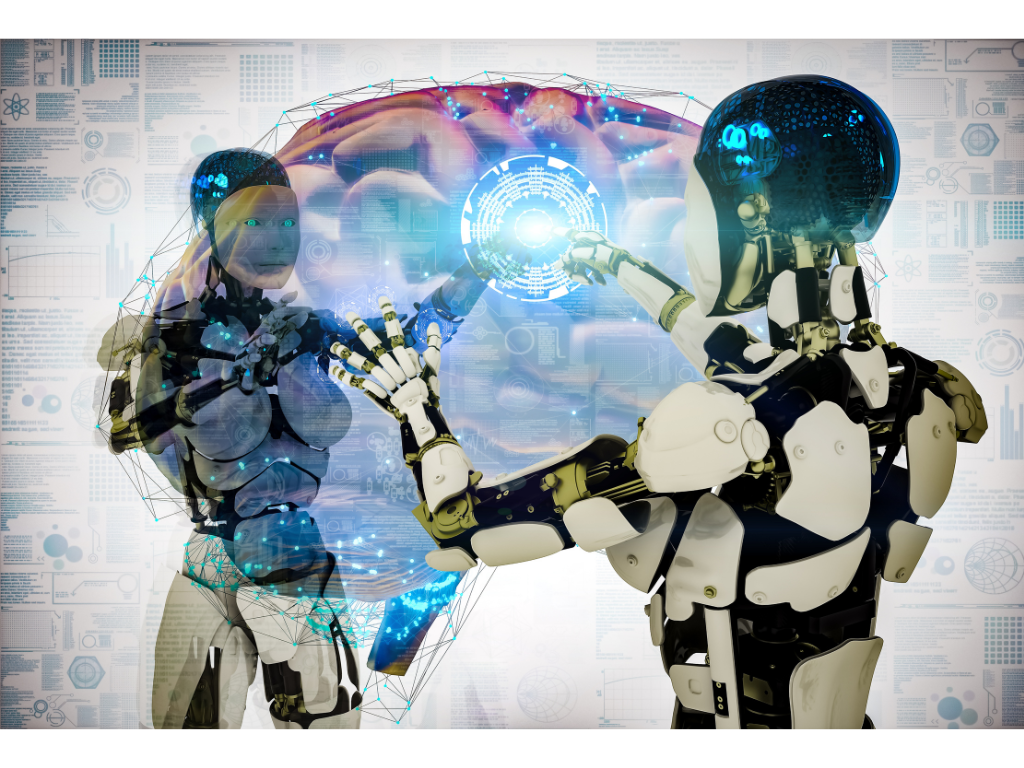
Published
How to Cite
Issue
Section
Categories
License
Copyright (c) 2022 Universidad Autónoma de Tamaulipas

This work is licensed under a Creative Commons Attribution-NonCommercial-ShareAlike 4.0 International License.